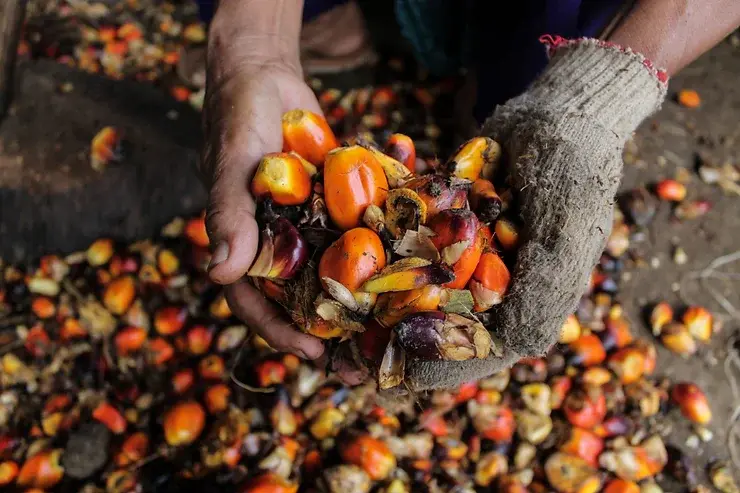
We continue today our Technology innovation journey with the use of Machine Learning model to increase crop yield
Background
Throughout the existence of agriculture, one of the main issues of interest to farmers was the issue of increasing yield. What are the best ways to increase yield per hectare? What are the factors that affect yield most? Recently, in view of the constant growth of the world’s population, this issue is becoming more and more relevant. However, with the emergence of new challenges for agrarians, there are also new ways and technologies appearing that are called to respond to them. This is what this article is about: what growers can do for increasing yield on their lands and what new technologies can help in this matter.
Palm oil is one of the largest commodities produced and needed in the world. 73 million metric tons were produced in 2020-2021 to meet the demand of the food, cosmetic, and fuel industries. Most of the fruit produced comes from Indonesia and Malaysia as the plant is suitable to grow in tropical areas. Having a huge amount of land for palm oil planting in East Kalimantan, PT REA Kaltim needs to handle their plantation carefully. REA Kaltim has over 75,000 hectares of land allocated for palm oil cultivation, in which more than 35,000 hectares are mature palm trees that continue to produce more than 900,000 tons fresh fruit bunch every year for the last four years.
Why Do We Need Yield Prediction?
Although REA Kaltim made a significant increase of palm oil production in 2018, their yield has been decreasing for the last three years. Usually expressed in Tons per Ha, Yield is considered to be probably the most important measure of performance, as it embodies the result of all the efforts and resources invested by agrarians in the development of plants in their fields.
To support Estate managers determining the underlying cause of poor yield based on relevant factors, early oil palm yield forecasting is essential. To ensure that current plantations contribute to the optimum yield possible without increasing land use, forecasting oil palm yield, and optimizing yield output per unit of land uses are crucial. Producing effective, quick, and accurate yield estimations under unexpected conditions of plantation and harvesting continues to pose a major challenge.
AI Machine Learning in Agriculture
Numerous studies and projects have been made to use new data science and analytics technology in agricultural sectors, one of them is Machine Learning. Machine learning is a subset of AI where machines are programmed to process and utilize data. In addition to efficient data gathering, machine learning aims to make use of the ever-growing amounts of data being gathered by modifying and analyzing them without major human input. Machine learning is a type of mathematical analysis that has a focus different from the typical analytical approach in applied subjects. In agriculture, machine learning is used in soil and water management, disease and pet control, crop quality control, and crop yield. One of the most important aspects of precision agriculture is yield prediction, which is crucial for mapping and forecasting yields, matching crop supply and demand, and managing crops to maximize productivity.
AI Machine Learning for Predicting Crop Yield
For the last decade, crop yield prediction using machine learning has already been studied with variants of algorithms, ranging from cereals to oilseeds, with temperatures, nutrients, and plant physiology as parameters. Kung et al. (2016) have studied tomato yield prediction using machine learning with the Ensemble Neural Network (ENN) method. The parameters they used were meteorological factors (e.g., relative humidity, precipitation, and air temperature), environmental factors (e.g., planting area, harvested area, harvest, and harvest per unit volume), and economic factors (e.g., the cost of production and the market trading price) from 1997-2014 in Taiwan. From this study, they found 3 models with error rate below 2% and two of the models with above 90% accuracy. Another study is predicting wheat yield in a 22-ha field in Bedfordshire, United Kingdom, has been done by Patanzi et al. (2015) using Supervised Kohonen Networks (SKN) that exceed 91% accuracy and subsequently reducing labor and time cost of soil sampling and analysis. We can assume from these two research that machine learning benefits the agricultural industry.
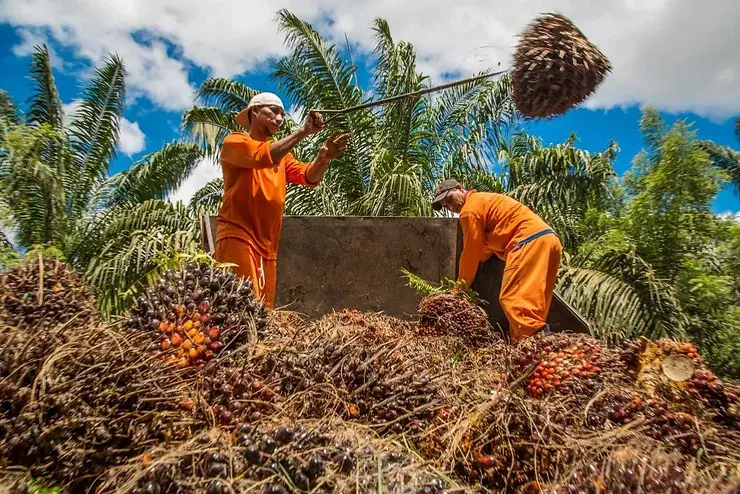
Our Project
BI Solusi Data Scientist built a task force with REA agronomist and other knowledgeable stakeholders to build a machine learning model that could predict Palm Oil plantation Yield.
Plant crop yield is dependent on a variety of variables, including climate, plant physiology, soil management, water usage, etc. First project step was to identify the variables that had the greatest impact on palm oil yield. We evaluate rain, planting material, maturing, and other attributes versus palm oil yield, while adding age as an additional attribute as it plays a significant role to the yield. We chose Random Forest as the model for this research as the parameters we use are non-parametric where the data we use do not depend on any distribution. Other than that, random forest has the major benefit of being applicable to both classification and regression problems, which make up most modern machine learning systems.
Results and Challenges
Our first attempt using rain, planting material, and maturing as attributes did not show any satisfactory results. The models we used did not show good regression, R-square values that the models produced are still below 0.5 and the attributes or parameters we used to predict crop yield are multicollinearity or when independent variables in the models are correlated, this shows that the models are still not reliable. Even though we have not yet found a model that fits properly, this research has improved once we modified the attribute to age, rainfall, and harvesting rotation. Despite the fact that, R-square value for harvesting vs. yield is already more than 0.9, we are still working to develop a better model for our client so that future predictions will be more precise.
We suspect that the data we used has flaws. Since studies have revealed a disparity between palm oil's potential and actual production. Considering numerous elements that must be considered while forecasting yield, like management of the plantation. For example, unexpected rain can prolong the harvesting interval, which will slow down the harvesting process. In addition, different harvesting/ techniques and whether the harvester performs plant recovery have an impact on crop yield in our client's vast plantations throughout East Kalimantan. Due to these reasons, we need to develop and explore more models that can accurately predict crop yield, and improve our methods of research.
We now know from our ongoing project that predicting is crucial for a process's productivity and efficiency, and we can use artificial intelligence to achieve that. Machine learning for projection is used in many industries, therefore we can assist you in improving and researching the forecasting for your own business. Please feel free to Contact us for our services; we will be your partner in technology and digital transformation!
BI Solusi is your trusted partner for data-driven success in Indonesia, serving companies in the Southeast Asia region and beyond. We specialize in implementing cutting-edge Data Analytics, Business Intelligence platform, and Big Data solution, complemented by expert Data Science services.
We offer flexible nearshore and offshore BI implementation models to meet your specific needs and deliver the highest-quality results.
Our BI Consulting expertise encompasses Data Integration services (ETL), Data Warehousing, and the utilization of Data Visualization tools such as Microsoft Power BI, Qlik Sense, and Tableau for Reports and Dashboards implementation.
Let us help you unlock the full potential of your data and achieve your business goals.
Comments