How NLP in BI Drives Conversational AI: Walmart's Secret to Superior Customer Service
- sitiatarfa8
- Apr 3, 2023
- 4 min read
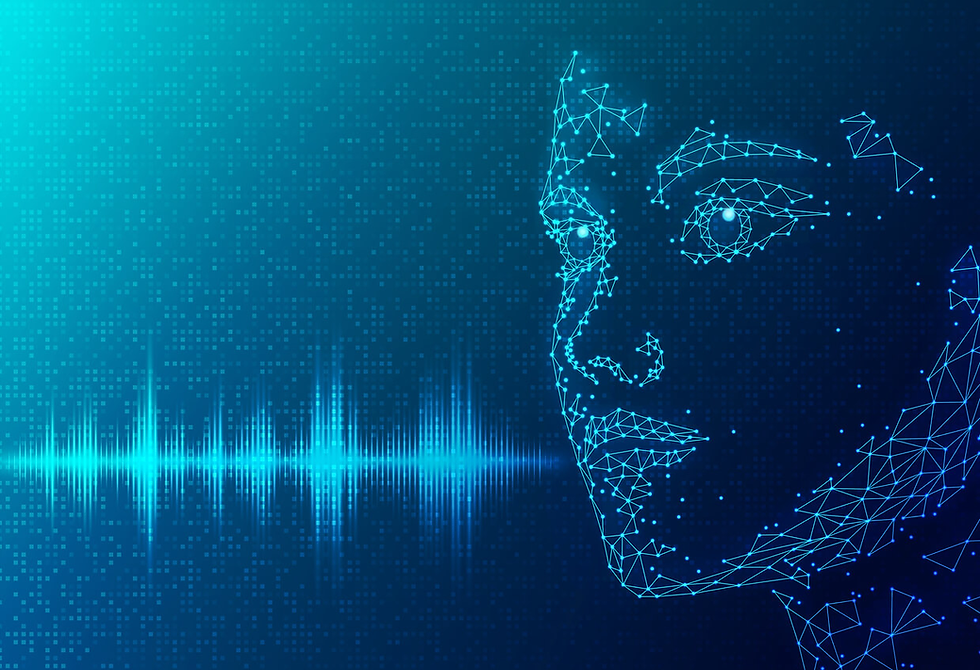
In most BI systems, data is accessed in a traditional way: logging into an application, generating the required report and filtering the insights through dashboards. But this often-lengthy process requires some technical proficiency. That means lower adoption rates. That’s why companies often resort to hiring data scientists and data analysts to extract insights from their BI systems. But managers also look for wider adoption within the organization. An increasing number of global companies are now adopting NLP-driven business intelligence chatbots that can understand natural language and perform complex tasks related to BI. Natural Language Processing (NLP) in Business Intelligence (BI) refers to the use of machine learning algorithms and computational linguistics to analyze and interpret human language data in order to gain insights and make better business decisions. NLP involves processing and analyzing large amounts of unstructured textual data, such as customer feedback, social media posts, online reviews, and internal communications, to identify patterns, trends, and other meaningful information that can help organizations improve their operations and outcomes.
NLP in BI typically involves several key steps, including data collection, text preprocessing (such as tokenization, stemming, and part-of-speech tagging), feature extraction, and modeling. NLP algorithms can be used for a wide range of tasks, such as sentiment analysis, topic modeling, named entity recognition, and text summarization.
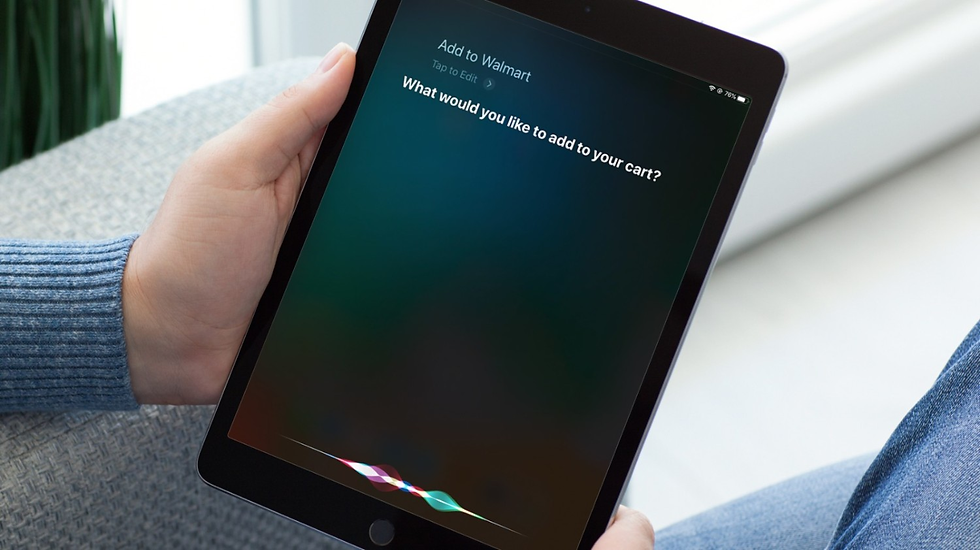
Use Case: Walmart
One example of a company that uses Natural Language Processing (NLP) in their Business Intelligence (BI) project is a customer service-focused organization, such as a Walmart Ask Sam.
By leveraging NLP in their business operation, Walmart can analyze customer interactions with their support team and derive insights into customer needs and preferences. For example:
Customer support: Walmart uses NLP to analyze customer feedback and inquiries across various channels, including social media, email, and online reviews. By analyzing this data, Walmart gains insights into customer sentiment, preferences, and pain points, allowing them to improve their products and services accordingly.
Inventory management: Walmart uses NLP to analyze customer purchase patterns and predict future demand for products. This allows Walmart to optimize their inventory levels and ensure that popular products are always in stock.
Product categorization: Walmart uses NLP to automatically categorize products on their website and mobile app. By analyzing the text descriptions of products, NLP algorithms can accurately categorize products into the appropriate categories, making it easier for customers to find what they are looking for.
Price optimization: Walmart uses NLP to analyze competitor pricing data and adjust their own prices accordingly. This allows Walmart to remain competitive while maximizing their profits.
Search optimization: Walmart uses NLP to improve their website and mobile app search capabilities. By analyzing customer search queries and product descriptions, NLP algorithms can provide more accurate search results, making it easier for customers to find what they are looking for.
Look into Walmart Ask Sam
The trend among customers is to move away from collaborative experiences to more autonomous ones, and AI is crucial in enabling them to offer such experiences at any time and place. As a result, Walmart has been building conversational AI capabilities over the past few years.
Using base Natural Language Understanding (NLU) capabilities, Walmart systems can quickly identify a customer's needs and context before executing the task. Text to Shop allows customers to search for items, add or remove products, reorder their favorites, and schedule a pickup or delivery through a quick text conversation using the same Conversational AI platform and retail AI models that power Voice Order.
Voice assistant for in-store associates, Ask Sam, enables customers to access store maps, look up prices, check messages, and more by verbally asking it questions. As shopping interfaces become more seamless and personalized, conversational AI plays a crucial role in improving customer and associate experiences across Walmart's business with millions of products and 230 million customers shopping each week.
Ask Sam has also been proven helpful for Walmart during the COVID-19 pandemic, highlighting the latest guidelines and dropping knowledge directly to users' fingertips—no typing required. And it will only become more sophisticated over time, leaning on its machine learning tech and a team of human engineers to improve accuracy. For an added layer of security, Ask Sam features an Emergency Alert Button, that empower managers to make quick decisions during high-stress situations. With the push of a button, clear and instructive emergency notifications are sent to all associates on and off the clock. That could mean the start of local lockdown procedures, moving employees and customers to a secure area inside the store, instructions to evacuate the facility, or a simple "all clear" to resume activities and report to shifts as normal.
Conclusion:
By leveraging NLP in BI, organizations can gain valuable insights into customer needs and preferences, identify emerging trends and opportunities, optimize their operations, and improve their overall performance. However, major challenges still exist to implementing NLP in BI, one is that bias against certain groups or demographics may be found in NLP models. Another is that while NLP systems require vast amounts of data to function, collecting and using this data can raise serious privacy concerns.
NLP models can also become more complex, and understanding how they arrive at certain decisions can be difficult. Therefore, it is essential to focus on creating explainable models, i.e., making it easier to understand how the model arrived at a particular decision.
Looking to take your business to the next level with the power of NLP? We've got you covered! Contact us now!
#NaturalLanguageProcessing #NLP #BusinessIntelligence #TextAnalytics #MachineLearning #ConversationalAI #AI
Comments